Ruisen Luo has recently published in his capacity of a corresponding author a research paper “Scalar Quantization as Sparse Least Square Optimization” in the top-notch journal of IEEE Transactions on Pattern Analysis and Machine Intelligence. SCU College of Electrical Engineering is the first work unit of this paper.
With an impact factor of 17.73 in 2018, and a 5-year impact factor of 16.887, IEEE Transactions on Pattern Analysis and Machine Intelligence is a top international journal in the fields of artificial intelligence, pattern recognition, image processing and computer vision. It is a tier-one academic journal of the Chinese Academy of Sciences, and a JCR top-notch journal. Google ranked it the No.1 most influential academic journal ( computer vision and pattern recognition ) in 2018. What’s more, it ranks the first place of all type A recommended journals in CCF artificial intelligence in terms of impact factor.
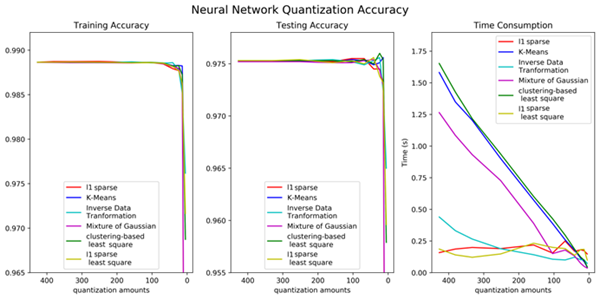
Vector quantization is an important method of signal compression. It can compress data to form a new vector on the premise of acceptable information loss by compressing the combination close to the original value. It has great practicability in speech recognition, image processing, machine learning, etc. “Popular clustering-based techniques suffers substantially from the problems of dependency on the seed, empty or out-of-the-range clusters, and high time complexity. To overcome the problems, scalar quantization is examined from a new perspective, namely sparse least square optimization. Specifically, several quantization algorithms based on l1 least square are proposed and implemented. In addition, similar schemes with l1+ l2 and l0 regularization are proposed. Furthermore, to compute quantization results with given amount of values/clusters, this paper proposes an iterative method and a clustering-based method, and both of them are built on sparse least square. The algorithms proposed are tested under three data scenarios and their computational performance, including information loss, time consumption and distribution of values of sparse vectors are compared. The paper offers a new perspective to probe the area of quantization, and the algorithms proposed are superior especially under bit-width reducing scenarios, when the required post-quantization resolution is not significantly lower than the original scalar.” ( Abstrac t)
University-Enterprise Cooperation Project 10.13039/ 501100001809 and National Natural Science of China have funded the research.
You may access the full paper at DOI: 10.1109/TPAMI.2019.2952096